SFR-RAG: Towards Contextually Faithful LLMs
Technical Report., 2024
SFR-RAG: Towards Contextually Faithful LLMs
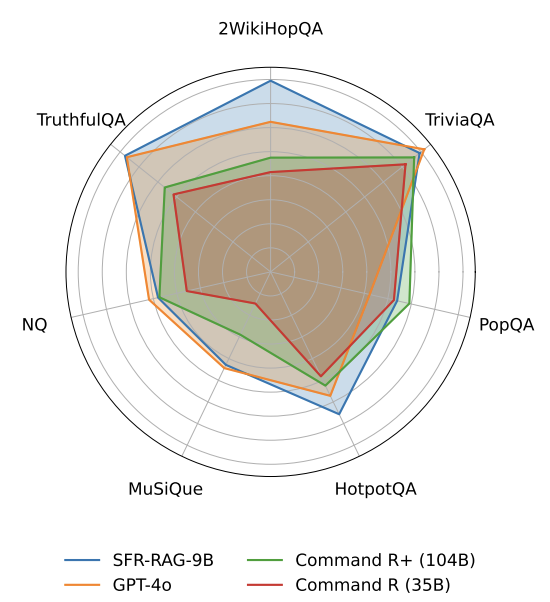
Citation: Xuan-Phi Nguyen, Shrey Pandit, Senthil Purushwalkam, Austin Xu, Hailin Chen, Yifei Ming, Zixuan Ke, Silvio Savarese, Caiming Xong, Shafiq Joty (2024). SFR-RAG: Towards Contextually Faithful LLMs. Arxiv Preprint - Technical report.
Paper Link: https://arxiv.org/abs/2409.09916